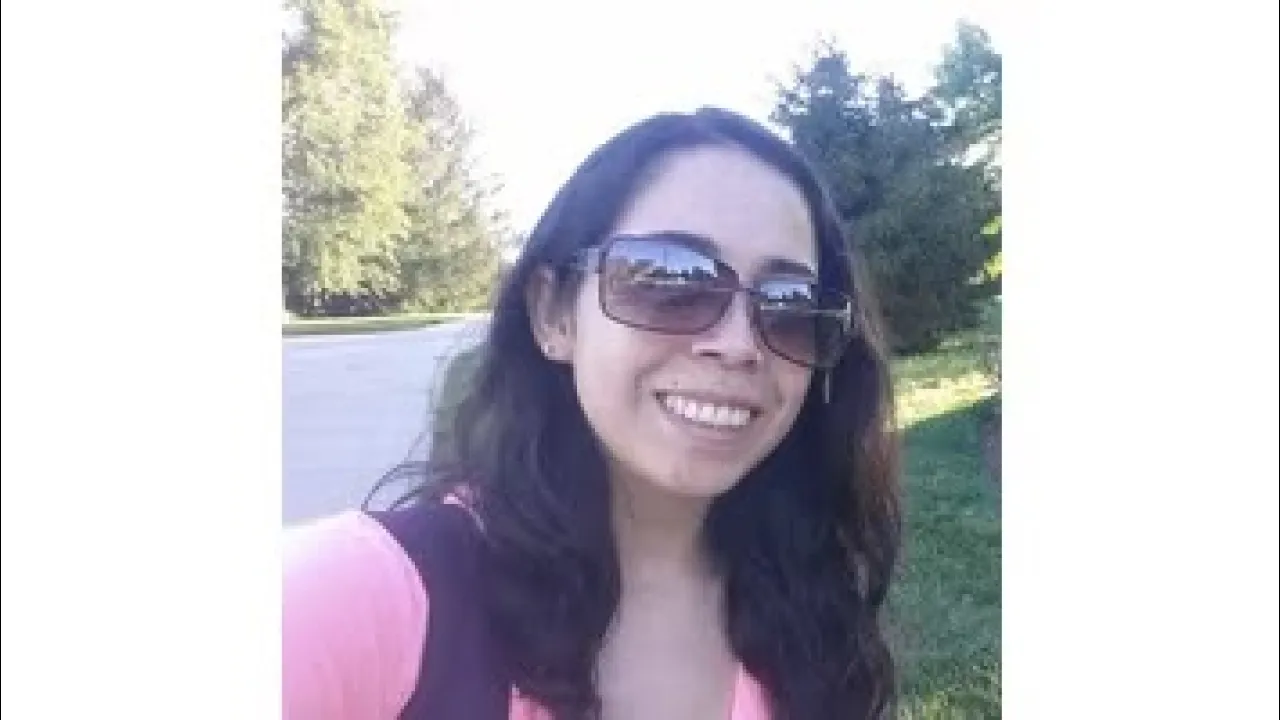
Block Nearest Neighbor Gaussian processes for large data
Overview
Abstract
We develop a valid spatial block-Nearest Neighbor Gaussian process (block-NNGP) for estimation and prediction of location-referenced large spatial data. The key idea behind the block-NNGP is to subdivide the spatial domain into several blocks which are dependent under some constraints. The cross-blocks capture the large-scale spatial variation, while each block capture the small-scale dependence. The block-NNGP is embedded as a sparsity-inducing prior within a hierarchical modeling framework. Markov chain Monte Carlo (MCMC) algorithms are executed without storing or decomposing large matrices, while the sparse block precision matrix is efficiently computed through parallel computing. Simulated examples illustrate the methodology, followed by concluding remarks.